서 론
소나무(Pinus densiflora Siebold & Zucc.)는 우리나라에 자생하고 있는 중요한 침엽수 중 하나로(Han et al., 1991), 경제적, 문화적, 환경적인 가치가 높다(Han et al., 2007). 따라서 소나무 형질개량과 육종연구는 중요한 과제이며 이를 위해 인위적으로 수분과 수정이 일어나게 하는 인공교배가 사용된다. 우리나라의 선발육종은 1950년대 말에 시작되었는데(Koo et al., 2006), 경제성이 있는 수종의 선발육종은 일반적으로 수형목 선발, 채종원 조성, 차대검정 과정 등을 거쳐 진행되며, 차대검정의 완료는 1세대 채종원의 유전간벌 또는 개량 채종원 조성으로 이어진다. 차대검정이 수행되는 수종의 진전세대 채종원이 조성되는 경우, 차대검정림의 개체를 이용할 수 있다(Kang et al., 2022).
소나무 1세대 채종원 조성은 앞서 언급된 전형적인 방법으로 진행되었다. Han and Kang(2004)에 따르면 1959년에 소나무 수형목 선발 및 지정 작업이 시작되었다. 개체선발의 결과로 다수의 소나무 수형목이 선정되었는데, Koo et al.(2006)과 Kim et al.(2019)은 수형목 선발 과정에 따라 총 425본의 소나무 수형목이 선발되었음을 적시했다. 선발된 수형목들은 1세대 채종원 조성에 이용되었으며(Jang et al., 2016). 소나무 2세대 채종원은 2016년부터 조성되었다(Park, 2015). 그 이전에 조성되어 2021년 12월 31일까지 유지되고 있는 소나무 1세대 채종원의 총 면적은 120.6 ha로, 지역별로는 강릉에 27.6 ha, 안면도에 77 ha, 춘천에 16 ha의 소나무 1세대 채종원이 분포하고 있다(Republic of Korea open government data portal, 2022).
차대검정과 채종원 개량도 일반적인 방법에 따라 진행되었다. Koo et al.(2006)은 1980년대에 여러 수종의 클론보존원 조성이 주로 이루어졌고, 소나무 클론보존원도 1980년대에 조성되었음을 밝혔다. Jang et al.(2016)에 따르면 소나무 수형목 413본에 대한 클론보존원이 조성되어 있다. 소나무 차대검정림에서 차대검정이 실시되었는데, 풍매 차대검정림을 이용하여 재적생장이 우수한 수형목이 식별되었으며, 인공교배 차대검정림을 바탕으로 재적생장이 우수한 교배조합이 식별되었다. 또한, 인공교배 조합을 이용한 2세대 채종원의 개량효과도 추정되었는데, 2세대 채종원은 1세대 채종원에 비해 재적생장 측면에서 약 7.5% 우수할 것으로 추정되었다. 이 때, 1970년대부터 2000년대까지 조성된 인공교배 차대검정림들이 연구에 이용되었다(Woo et al., 2016). 연구결과를 바탕으로 채종원 개량이 이루어졌는데, Jang et al.(2016)에 따르면 풍매 차대검정림의 차대검정 결과를 바탕으로 1세대 채종원의 유전간벌이 실시되었고 인공교배 차대검정림의 차대검정 결과를 바탕으로 2세대 채종원이 조성되었다.
식물의 인공교배는 교배봉투를 이용해 불필요한 화분의 수정을 막고, 실험자가 원하는 화분을 수정시키는 작업이다(Allaby, 1998). 인공교배 과정은 화분 채취 및 저장, 교배봉투 씌우기, 인공수분, 교배봉투 제거와 해충관리로 구성된다. 이 때, 암꽃이 피기 전, 암구과가 완전히 성숙하기 전에 미리 교배봉투를 씌워야 하며, 암수한몸인 수종의 경우 교배봉투를 씌우기 전에 수꽃을 제거(제웅)해야 한다(Kang et al., 2022).
인공교배는 교잡육종, 집단의 개량, 유전적 분석 등에 사용된다. Colombo and Galmarini(2017)는 채소종에서 교잡으로 만들어진 종자의 품질을 보장하는데 인공교배가 중요함을 역설하였으며, Lee and Kim(2007)은 인공교배를 이용한 인위적 종간 교잡이 임목 신품종 육성에 중요하다는 점을 강조하였다. 또한, Li and Weir(1999)는 인공교배를 통해 임목 차대의 유전획득량을 극대화할 수 있다고 밝혔다. 유전체 선발(Genomic selection)에서도 인공교배가 이용될 수 있는데, Mphahlele et al.(2020)은 genomic selection에서 인공교배를 이용하여 자배 억제와 집단 개량을 동시에 달성할 수 있다고 주장하였다. 인공교배를 이용하면 유전학 연구에도 도움을 받을 수 있다. 몇 가지 예를 들 수 있는데, Lynch and Walsh(1998)는 유전공분산을 구하는 과정에서 반형매 차대는 상가적 효과만을 확인할 수 있고 전형매 차대를 이용하는 경우 상가적 효과와 함께 우성 효과도 확인할 수 있다는 점을 밝혔다. 또한, Liu(1998)는 인공교배를 통해 만들어진 집단과 자연집단에서의 QTL mapping에 대해 언급하였는데, 인공교배를 이용한 경우의 통계적 검정력이 더 높다고 보고하였다.
인공교배 과정에는 화분오염이라는 문제점이 존재한다(Tan et al., 2018). 인공교배에서의 오염은 여러 원인으로 발생할 수 있는데, 꽃 또는 구과의 격리가 제대로 되지 않거나 목적하지 않는 화분을 수집하여 인공수분 시켰을 때 오염이 나타날 수 있다(Vidal et al., 2015). 장소 역시 오염에 영향을 미치는 인자일 수 있는데, Horsley et al.(2010)은 야외에서 인공교배를 할 때의 화분오염률이 온실에서의 오염률보다 높을 수 있다고 보고하였다.
Madesis et al.(2013)에 따르면 microsatellite marker는 반복되는 서열로 구성되는 marker를 뜻하며, 일반적으로 microsatellite의 반복 단위는 1~6개의 염기로 정의된다. CA가 17번 반복되는 서열((CA)17)이 microsatellite의 한 예시이다(Queller et al., 1993). Microsatellite는 short tandem repeats (STRs), simple sequence repeats (SSRs), simple sequence length polymorphisms (SSLPs)로 불리기도 한다(Yun et al., 2011). 최근에는 SNP marker의 사용이 늘어나고 있는 추세이지만, microsatellite는 멘델 유전양상을 따르고, 대립유전자의 다형성이 있다는 장점을 가져 혈통분석(pedigree analysis)에 사용되어왔다(Flanagan and Jones, 2019). 최근에도 microsatellite를 이용한 연구들이 진행되고 있다(Willows-Munro and Kleinhans, 2020; Ye et al., 2020). 나무를 대상으로 한 예시도 몇 가지 있는데, de Oliveira et al.(2023)은 포도 잡종(hybrids of Vitis spp.)의 부모 식별을 위해 21개의 microsatellite를 사용했다. Moreno-Sanz et al.(2020)은 올리브(Olea europaea L.) Casaliva 품종의 배아를 대상으로 화분수를 확인했는데, 이 때 11개의 microsatellite를 이용한 혈통분석을 이용했다. Žulj Mihaljević et al.(2020)은 재배종 포도(Vitis vinifera L. subsp. sativa) 품종의 혈통분석을 위해 20개의 microsatellite를 사용했으며, 혈통분석을 바탕으로 품종 간 친족관계(kinships)도 확인했다. 따라서 혈통분석 연구에서 microsatellite는 유용하게 사용되고 있다고 판단할 수 있다.
침엽수의 인공교배 효율을 microsatellite를 바탕으로 평가, 분석한 연구도 존재한다. Sun et al.(2017)은 잎갈나무(Larix gmelinii var. principis-rupprechtii (Mayr) Pilg.) 채종원 인공교배 효율을 평가했으며 Grattapaglia et al.(2014)은 테다소나무(Pinus taeda L.) 채종원의 인공교배 효율을, Kumar et al.(2007)은 라디아타소나무(Pinus radiata D.Don)의 인공교배 효율을 고찰했다.
본 연구에서는 microsatellite를 이용한 혈통분석 연구를 소나무 인공교배 집단에 적용하여 인공교배 효율 및 차대집단의 관리상황을 평가하고자 하였다. 특히, 이번 연구 대상인 인공교배 집단을 조성할 당시에 평년보다 높은 기온으로 교배시기를 정확히 맞추기 어려웠으며, 강풍으로 인해 교배봉투가 찢어지는 등의 문제가 존재했다. 임목은 생장기간이 길다는 특징을 가지기 때문에(Kang et al., 2022), 파종 이후부터 실제 연구에 사용되기까지 긴 시간이 소요될 수 있어서 파종 후 실제 연구에 사용될 때 까지의 기간 동안 다양한 관리 문제 가능성이 존재한다. 인공교배는 진전세대 채종원 조성 및 임목의 유전 연구에 사용되는 중요한 기법인 만큼, 이번 연구를 통해 인공교배 과정 및 인공교배 이후 육종집단 관리 후 부모가 확실한 차대 개체를 어느 정도 생산해낼 수 있는지, 또한 주의할 사항은 무엇인지 고찰해보고자 한다.
재료 및 방법
본 연구는 소나무 인공교배 차대 집단 5년생 개체를 대상으로 수행되었다. 인공교배는 1977년에 조성된 충남 안면도 소나무 채종원에서 2015년 5월 6일과 7일에 진행되었다. 교배모수와 화분수로는 충북2호와 강원40호가 이용되었다. 인공교배는 두 방식으로 진행되었는데, 충북2호를 모수로, 강원40호를 화분수로 하는 인공교배(충북2호×강원40호)와 강원40호를 모수로, 충북2호를 화분수로 이용한 인공교배(강원40호×충북2호)를 수행하였다. 인공교배로 만들어진 구과들은 2016년 9월 20일에 채취했다. 또한, 구과에서 충실종자를 선별한 뒤 저온저장을 거쳐 2017년 4월 서울대학교 칠보산 학술림에 파종하였다.
조사 당시 총 159개체가 생존하고 있었으며, 개체목 인식표가 중복되는 오류가 확인되어 실험재료를 재 분류하여 실험을 실시하였다. 159개체는 충북2호×강원40호 교배조합만 존재하는 소집단(이하 소집단 1)과 충북2호×강원40호와 강원40호×충북2호 교배조합이 섞여 있는 것으로 추정되는 소집단(이하 소집단 2)으로 구별되었다. 소집단 1은 96개 개체로 구성되며, 소집단 2는 63개 개체로 구성되었다. 소집단 1에 속하는 개체목의 인식표는 다른 158개의 개체목 인식표와 중복되지 않았다. 반면, 소집단 2에 속하는 개체목들 중 60개 개체에서 30쌍의 동일한 인식표가 확인되었으며 나머지 3개 개체의 인식표가 동일했다.
원래 충북2호×강원40호 집단과 강원40호×충북2호 집단은 개체목 인식표에 집단 번호를 사용하여 구별했다. 충북2호×강원40호 집단은 3으로 표기하였고, 강원40호×충북2호 집단은 2로 표기했다. 예를 들어, 3-44-10은 충북2호×강원40호 개체이며 2-44-10은 강원40호×충북2호 개체이다. 한편, 중복되는 인식표를 가진 개체목들의 경우 알파벳으로 개체들을 구분하였다. 예를 들어, 3-44-10로 표기된 차대목이 두 개 발견되어 두 개체를 각각 3-44-10a, 3-44-10b로 나누었고, 3-47-8의 경우 중복되는 개체목 인식표가 세 개 존재하여 따라서 이를 3-47-8a, 3-47-8b, 3-47-8c로 구분했다.
DNA 추출을 위한 잎 샘플은 2021년 7월 30일과 2022년 2월 9일에 서울대학교 칠보산 학술림 현지에서 채취되었다. 잎 샘플을 아이스박스에 넣어 실험실까지 운반했으며, 실험실 도착 후 −20°C 냉장고에 보관하였다.
DNA는 DNeasy® Plant Mini Kit (QIAGEN)과 GeneAll® ExgeneTM Plant SV kit (GeneAll)를 이용하였으며, 추출 과정은 각 회사의 메뉴얼을 따랐다. 잎 조직을 분쇄하기 위해 TissueLyser II (QIAGEN)를 사용하였다. 분쇄 과정은 frequency 초당 30회, 1.5분 조건으로 lyser adapter의 방향을 달리하여 두 번 진행되었다. 잎을 분쇄하기 전부터 분쇄된 잎에 kit의 buffer를 처음 사용하기 전까지 액체질소를 이용하여 낮은 온도를 유지하였다. 추출이 완료된 뒤 Nanovue Plus spectrophotometer with printer (GE healthcare)를 이용하여 DNA의 농도와 순도를 측정했다.
Microsatellite 프라이머는 Chung et al.(2019)이 소나무에 대해 개발한 19개 프라이머 중 Lee et al.(2021)이 인공교배 F1 집단(충북2호×강원40호 및 강원40호×충북2호)과 충북2호, 강원40호의 친자관계를 확인하기 위해 선별한 6개 프라이머(CPDE 0039, CPDE 0057, CPDE 0076, CPDE 0077, CPDE 0106, CPDE 0137)를 사용하였다(Table 1). Lee et al.(2021)은 충북2호, 강원40호, 충북2호와 강원40호의 친자 혈통으로 확인된 차대목들을 이용하여 증폭 결과가 좋고 친자 관계를 잘 설명하는 프라이머들을 선별했다. 따라서 충북2호, 강원40호와 차대목의 친자 관계에서 나타날 수 있는 microsatellite 대립유전자들을 증폭하는 프라이머들이 선별되었다. 연구에 사용된 프라이머의 증폭산물 크기 범위(size range)가 인공교배 집단의 microsatellite 단편(fragment)들의 길이를 포괄하지 않아서 이를 수정하여 사용했다.
Size ranges and the Tm of this table is different from original values from Chung et al.(2019).
PCR을 위해 추출된 DNA 용액을 5 ng/μl로 희석하였다. 반응용액은 DNA 4.00 μl, 3차 증류수 8.60 μl, 10X reaction buffer (BIOFACT) 1.60 μl, 10mM dNTP (BIOFACT) 0.32 μl, A-Star Taq DNA polymerase (BIOFACT) 0.20 μl (5 unit), forward/reverse primer (Macrogen) 각 0.32 μl, M13 primer (Macrogen) 0.64 μl로 구성되었다. Bioer LifePro Thermal Cycler (Bioer Techonology)를 이용하여 PCR을 진행하였다. 5분, 95°C조건에서 초기 열변성을 한 다음, 30초, 95°C 열변성(denaturing), 30초 56°C 어닐링(annealing), 1분 72°C 증폭(extension)을 10회 실시하였다. 그 다음 30초, 95°C 열변성, 30초 52°C 어닐링, 1분 72°C 증폭을 진행한 뒤 10분, 72°C에서 추가 반응을 하였다.
PCR 반응이 끝난 시료는 ABI 3730xl Genetic Analyzer (Life Technologies™)를 이용하여 단편 분석을 진행하였다. 그 뒤 Geneious Prime® ver.2021.2.2. (Biomatters, https://www.geneious.com)를 이용하여 각 샘플의 유전자형을 확인하였다. Null allele, stuttering과 large allele dropout을 확인하기 위해 Micro-Checker ver.2.2.3 (Van Oosterhout et al., 2004) 프로그램을 이용하여 분석을 진행하였다. 그 뒤 연구에 사용된 Microsatellite 프라이머 개수의 적절성을 평가하기 위해 GenAlEx 6.51b2 (Peakall and Smouse, 2006)와 GenClone 2.0 (Arnaud-Haond and Belkhir, 2007)을 이용하였다. GenAlEx 6.51b2를 통해 PI 값과 PIsib 값을 산출하였고, GenClone 2.0을 사용해 Psex값을 도출하였다. 유전자형의 누락이 있는 경우에는 GenClone 2.0에서 Psex를 산출할 수 없으므로, 부득이하게 유전자형 누락이 있는 개체를 제외하고 분석을 진행했다.
마지막으로, 혈통분석 결과에 대한 통계검정을 위해서 CERVUS ver.3.0.7 (Kalinowski et al., 2007)을 이용했다. CERVUS를 이용한 분석은 allele frequency analysis, simulation of parentage analysis, parentage analysis 순서로 진행했다. Simulation of parentage analysis 단계에서 number of offspring 값은 100,000, proportion of candidate mothers sampled 값은 0.4726을 사용했다. Maternity analysis를 위한 simulation에서는 number of candidate mothers 값은 2로 하였고, test for self fertilization 옵션을 적용시켰다. Parent pair analysis (sexes known)를 위한 simulation에서는 number of candidate parents 값을 2로 설정했으며, proportion of candidate mothers sampled와 proportion of candidate fathers sampled 값은 0.4726을 사용했다. 두 분석 모두에서 proportion of loci typed 값은 0.99, proportion of loci mistyped 값은 0.01, 그리고 minimum number of typed loci 값은 5로 하였다. Delta 값을 이용해 simulation을 진행했으며 신뢰 수준은 95%와 80%를 사용했다. Parent pair analysis를 할 때 maternity analysis에서 모수가 충북2호도, 강원40호도 아닌 것으로 식별된 개체는 제외하였다. Parent pair analysis 결과, LOD 값이 0을 넘더라도 delta 값이 0으로 나오는 문제가 발생하여, 산출된 LOD 값과 critical delta 값을 이용해 직접 분석을 진행했다.
육종집단 관리 문제의 가능성이 있는 54개의 차대목을 제외한 뒤 나머지 105개체를 이용해 같은 분석을 다시 진행하였다. 2차 혈통분석은 1차 혈통분석과 달리 simulation of parentage analysis 과정에서 proportion of candidate mothers sampled, proportion of candidate fathers sampled 값을 0.7142로 설정하여 실시했다.
결과 및 고찰
인공교배 차대묘 총 159본에 대하여 Micro-Checker ver.2.2.3을 이용하여 분석한 결과 PCR에서의 large allele dropout이 존재하지 않았으며, DNA polymerase의 slippage에 의해 만들어진 stutter sequence도 없었다. CPDE0077 프라이머에서 null allele이 존재하는 것으로 추정되었는데(p<0.001), 다른 다섯 개 프라이머에서는 null allele이 없는 것으로 확인되었다(p>0.05).
GenAlEx 6.51b2에 의해 PI 값은 1.8×10−5, PIsib 값은 9.3×10−3으로 산출되었다. PI는 임의의 두 개체가 같은 유전자형을 가질 확률, PIsib은 임의의 두 형제자매가 같은 유전자형을 가질 확률이다. PI 값과 PIsib 값은 유전자형을 이용해 개체들을 식별하는데 필요한 유전자좌의 개수를 판단할 때의 기준으로 사용될 수 있다(Waits et al., 2001). 본 연구에서 확인된 PI 및 PIsib은 여러 연구에 비해 높은 값을 보인다(Eusemann and Liesebach, 2021; Zádrapová et al., 2020; D’Amico et al., 2019; Tarroux et al., 2014; Hasenkamp et al., 2011).
본 연구의 PI와 PIsib 값이 선행논문에 비해서 높지만, 연구에 사용된 프라이머의 수(6개)는 적절하다. D’Amicoet al.(2019)은 3×10−3의 PIsib 값을 제시하면서, 연구에서 사용된 프라이머의 수(9개)가 개체 식별에 사용 가능한 수준이라고 주장했다. 유럽너도밤나무(Fagus sylvatica L.) Hilchenbach 집단의 경우 상대적으로 높은 PIsib 값을(4×10−3)가졌지만, 해당 집단에서 유전자형이 동일한 개체들은 모두 근연관계에 속했고, PI 값이 1.6×10−7였기 때문에 6개 프라이머를 이용해도 식별력이 충분했다(Hasenkamp et al., 2011). Waits et al.(2001)은 자연집단에서 개체들을 식별할 때 PI 값이 1×10−3-1×10−4정도면 충분하다고 언급했다.
본 연구의 PIsib 값은 9.3×10−3으로 D’Amico et al.(2019)의 결과와 같은 10−3규모의 값이다. PI 값의 경우 1.8×10−5으로 Waits et al.(2001)이 제시한 기준에 부합한다. 본 연구는 자연집단을 대상으로 이루어지지는 않았기에 Waits et al.(2001)의 결론을 그대로 사용하기에는 어려울 수 있지만 본 연구의 PI 값이 1×10−4보다 작은 값이었다는 점과 유전자형이 동일한 차대묘(총 14쌍)들은 모두 근연관계에 있었다는 점을 모두 고려한다면, 본 연구의 PI 값도 적정 수준이라고 판단할 수 있다.
Waits et al.(2001)이 언급했던 자연집단과 달리 본 연구는 인공교배 집단을 대상으로 했으므로 Psex값을 통해 프라이머 수의 적절성을 추가적으로 평가했다. GenClone 2.0에 의해 도출된 Psex값은 모두 0.05보다 작았다. Psex값은 유전자형이 같은 개체가 존재할 때만 산출되는 값으로 0.05보다 작은 Psex값은 연구에 사용된 프라이머들을 바탕으로 클론 다양성에 대한 명확한 추정이 가능함을 의미한다(Arnaud-Haond et al., 2005). GenClone 2.0을 클론이 아닌 집단의 연구에도 사용 가능하기에(Arnaud-Haond and Belkhir, 2007) 이번 연구에 Psex값을 적용 가능하다. PI 값, PIsib 값, Psex 값의 적절성을 토대로, 본 연구에 사용된 프라이머 수(6개)는 적절하다고 할 수 있다.
CERVUS ver.3.0.7을 이용한 maternity analysis 결과에서(Table 2) critical delta 값은 95% 신뢰수준에서 1.26, 80% 신뢰수준에서 0.00이었다. 95% 신뢰수준에서 모수가 충북2호 또는 강원40호인 개체는 총 55개(34.6%)였으며, 80% 신뢰수준에서 모수가 충북2호 또는 강원40호인 개체는 총 126개(79.2%)였다. CERVUS ver.3.0.7은 LOD 값을 이용해 차대 개체별로 모수일 가능성이 가장 높은 후보를 산출한다. CERVUS ver.3.0.7결과 후보 모수 중 가장 가능성이 높은 모수 개체와 유전자형의 불일치가 있는 3개(1.9%)의 차대목들은 모수가 충북2호도, 강원40호도 아닌 것으로 나타났다. 또한, 강원40호가 모수일 가능성이 가장 높은 개체 중 30개(18.9%)의 차대목들도 강원40호가 모수가 아닌 것으로 추정되었다. 모수가 충북2호 또는 강원40호로 추정된 모든 개체들은 추정된 모수와 유전자형의 불일치가 없었다.
강원40호와 유전자형의 불일치가 없지만 강원40호가 모수가 아니라고 식별된 30개 차대목의 경우, 통계 분석과는 달리 실제 모수가 강원40호일 가능성도 존재한다. 이를 위해 CERVUS ver.3.0.7에서 사용하는 계산식을 확인할 필요가 있다. 화분수의 유전자형을 모르는 경우 maternity analysis에 사용되는 LOD 계산식은 다음과 같다.
이 때 P(gm)과 P(go)는 하디-바인베르크 원리를 가정했을 때의 모수와 교배 차대목의 유전자형 빈도이다. T(go|gm)는 조건부 확률의 일종으로, 모수 유전자형을 가정했을 때 멘델 유전에 의해 교배 차대목 유전자형이 나타날 확률이다. ε는 유전자형 식별의 오류 확률이다(Kalinowski et al., 2007).
충북2호의 경우 CPDE0137에서만 동형접합자이며 강원40호의 경우 CPDE0076에서만 동형접합자이다. 따라서 모수와 교배 차대묘가 모두 이형접합자인 경우를 예시로 살펴볼 수 있다. 예를 들어, 모수의 유전자형이 AB, 교배 차대목의 유전자형이 BC라고 하고, 대립유전자 A, B, C의 대립유전자 빈도가 a, b, c라고 하자. 이 때 T(go|gm)값은 모수가 B를 물려줄 확률(1/2)과 화분수에서 대립유전자 C가 전해지는 확률(c)의 곱인 c/2가 된다(Marshall et al., 1998). P(go)는 하디-바인베르크 원리에 의해서 2bc가 된다.
LOD 계산식에 따르면 T(go|gm)보다 P(go)의 효과가 더 큰 경우 LOD 값이 음수로 나타날 수 있다. LOD 계산식에서 P(gm)P(go)로 진수의 분자와 분모를 나누면 T(go|gm)이 P(go)보다 큰 경우 LOD 값이 양수가 되며, T(go|gm)이 P(go)보다 작은 경우 LOD값이 음수가 되는 것을 알 수 있다(Additional file 1). 위 예시에서 c/2 값이 2bc 보다 작으면 LOD 값이 음수가 된다. 즉, b의 값(B 대립유전자의 대립유전자 빈도)이 1/4 보다 큰 경우 LOD 값이 음수가 된다.
본 연구는 인공교배 차대묘 집단을 대상으로 진행되었으며, 모수 후보와 유전자형 불일치가 없는 차대묘가 전체의 98.1%에 달했다. 따라서 충북2호와 강원40호가 가지고 있는 대립유전자의 경우 대립유전자 빈도가 높게 나타난다. 따라서 위의 예시와 같이 하나의 대립유전자를 공유하는 모수와 교배 차대묘가 존재할 때, 이형접합 모수가 이형접합 교배 차대묘에 물려준 대립유전자의 빈도가 1/4 보다 높은 경우가 존재한다. 이와 더불어, 모수와 유전자형 차이가 존재하지 않는 선에서 LOD 값을 음수로 만드는 조건들(Additional file 2)이 강하게 나타난 차대목의 경우 통계분석에서 강원40호가 모수가 아닌 것으로 나타날 수 있다.
위음성(false-negative)의 가능성이 있지만, 통계적으로 충북2호 또는 강원40호가 모수인 것으로 식별된 차대묘만 이용하여 parent pair 분석을 실시했다(Table 3). Critical delta 값은 95% 신뢰수준에서 3.86, 80% 신뢰수준에서 0.00이었다. 126개 개체 중 60개(47.6%) 개체가 충북2호와 강원40호의 친자 혈통으로 식별되었다. 또한, 8개체(6.3%)가 충북2호, 2개체(1.6%)가 강원40호의 자배로 만들어진 개체로 추정되었다. 충북2호와 강원40호의 친자 혈통으로 추정된 차대묘 중 하나(3-44-10b)는 강원40호와 유전자형의 불일치가 1개 존재했다. 충북2호의 자배로 만들어진 것으로 추정된 차대묘 중 하나(3-46-2b)는 충북2호의 자배를 가정했을 때 유전자형의 불일치가 1개 존재했으며, 두 개(3-45-5a, 3-54-4)는 충북2호의 자배를 가정했을 때 유전자형의 불일치가 2개 존재했다.
The values in bracket are about all samples, the values out of bracket don’t contain samples which mother tree is neither CB2 nor KW40.
첫 번째 parent pair analysis 결과(Figure 1) LOD score가 0을 넘는 차대묘는 60본이었으며, LOD score가 0이하인 차대묘는 66본이었다. 이 때 LOD score가 0을 넘는 차대묘들 모두 delta 값이 0을 넘었다.
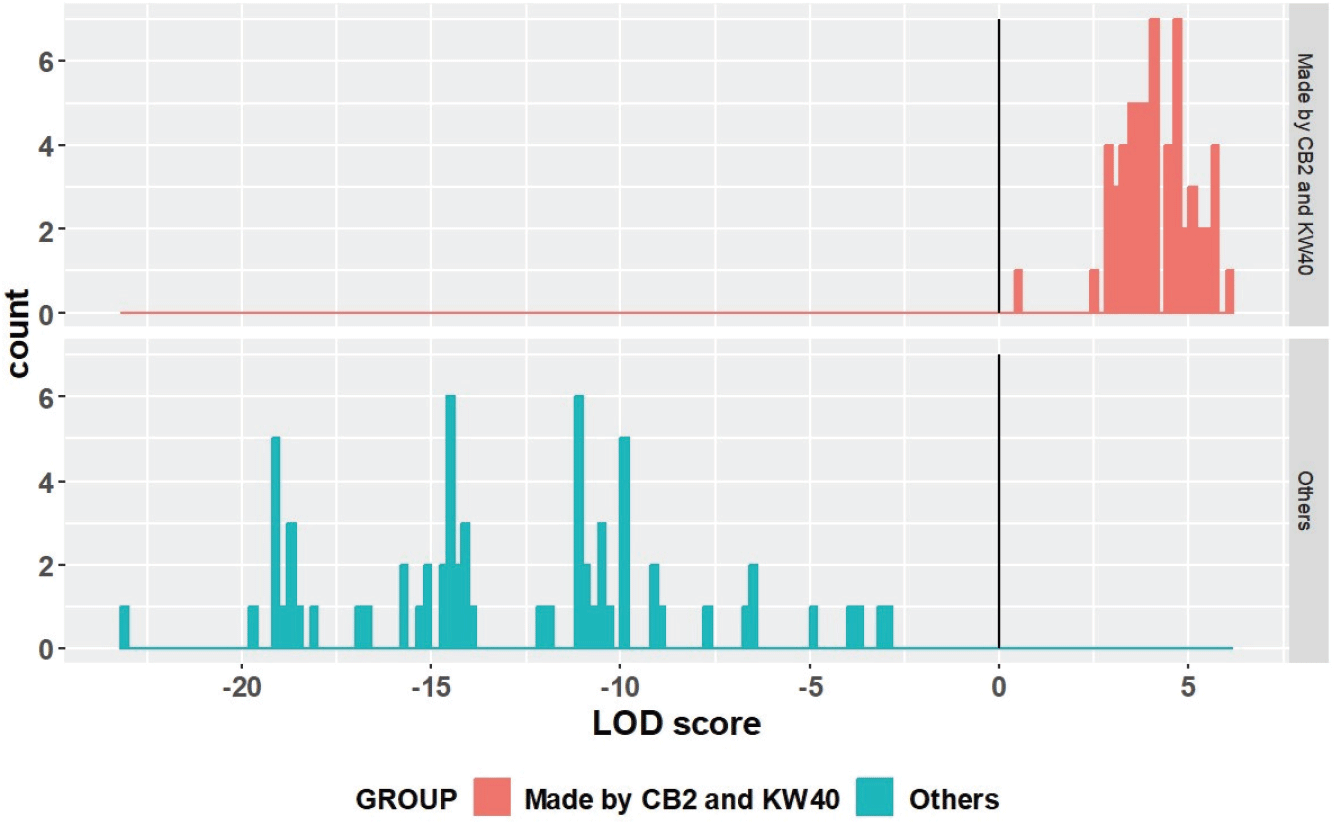
소집단 2에서 중복되는 개체목 인식표를 가진 개체가 세 개체 존재했다. 앞서 밝힌 바와 같이, 충북2호×강원40호 집단과 강원40호×충북2호 집단이 섞이는 외의 다른 문제가 없다면 중복되는 개체목 인식표는 세 개 이상일 수 없다. 3-47-8의 경우, 같은 개체목 인식표가 세 개 있었는데 이를 통해 관리상의 문제점이 있었다고 판단할 수 있다.
Maternity analysis 결과에서도 문제가 나타났다. 소집단 1에 속하는 차대목 중 모수가 강원40호로 추정된 개체가 35개 존재했다. 또한, 소집단 2에서는, 중복되는 인식표를 가지는 개체들이 모수가 같은 경우가 존재했다. 예를 들어, 3-45-12a와 3-45-12b 모두 모수가 강원40호로 식별되었다. 앞서 말한 바와 같이, 중복되는 개체목 인식표를 가지는 차대목의 각 모수는 서로 다르다. 따라서 이론적으로는 모수가 같을 수가 없다(Table 4). Grattapaglia et al.(2014)에 따르면 채종원 조성 당시의 클론명 오표기에 의해 이런 현상이 발생할 수 있다. 채종목의 클론명 오표기의 가능성도 있지만, 동일한 인식표를 공유하는 세 개체가 존재한다는 점을 고려했을 때, 관리상의 문제 가능성을 배제할 수는 없다. 따라서 이와 같은 문제가 존재하는 경우(전체 159본 중 54본)를 제외하고 다시 분석을 실시했다.
One out of a labeling pair (with a) (e.g. 3-45-12a) | One out of a labeling pair (with b) (e.g. 3-45-12b) | |
---|---|---|
Possible mother tree combinations | CB2 | KW40 |
KW40 | CB2 |
In case of 3-47-8a, 3-47-8b and 3-47-8c, the existence of three 3-47-8 labeling is a problem itself.
이 때, 관리상의 문제 외에 다른 이유로 위와 같은 상황이 발생할 수 있으므로 주의가 필요하다. 또한 통계 분석에 따라 지정된 모수가 개체목 인식표로 예상 가능한 모수와 같은 경우에도 아무 문제가 없다고 단정할 수 없다. Adams et al.(1988)는 인공교배의 에러가 발견되지 않더라도 교배상의 문제가 있을 수 있음을 지적했는데, 화분오염으로 만들어진 차대목의 유전자형이 우리가 목적한 화분수를 바탕으로 예측 가능한 유전자형에 부합할 가능성이 있다. 따라서 친자 혈통으로 확인된 차대목 중에서도 화분오염으로 만들어진 개체들이 존재할 수 있다. 또한, 관리상의 문제점이 있었지만 충북2호와 강원40호의 유전자형을 바탕으로 예상 가능한 친자 혈통의 유전자형이 나타날 가능성도 존재한다.
인공교배 차대묘 105본에 대하여 Micro-Checker ver.2.2.3을 이용하여 분석한 결과 PCR에서의 large allele dropout이 존재하지 않았으며, DNA polymerase의 slippage에 의해 만들어진 stutter sequence는 없었다. CPDE0077 프라이머에서 null allele이 존재하는 것으로 추정되었는데(p<0.001), 다른 다섯 개 프라이머에서는 null allele이 없는 것으로 추정되었다(p~0.05).
GenAlEx 6.51b2에 의해 PI 값은 1.4×10−5, PIsib 값은 8.9×10−3으로 산출되었다. 이 값은 D’Amico et al.(2019)의 PIsib 값인 3×10−3과 함께 10−3규모의 값이다. 또한, PI 값은 1.4×10−5로 Waits et al.(2001)이 제시한 기준(1×10−3-1×10−4)보다 낮으며, 유전자형이 동일한 차대묘(총 7쌍)들은 모두 근연관계에 있었다. 따라서 본 연구의 6개 프라이머는 Hasenkamp et al.(2011)이 유럽너도밤나무 Hilchenbach 집단에 적용한 6개 프라이머와 비슷한 조건에 해당된다.
1차 혈통분석과 마찬가지로, Waits et al.(2001)이 자연집단에 대한 기준을 제시했다는 문제점이 존재한다. 따라서 Psex값을 이용한 프라이머 수의 적절성 평가를 진행했다. Genclone 2.0에 의해 산출된 Psex값은 모두 0.05보다 작았다. 따라서 본 연구에서 6개 프라이머를 사용한 것은 적절하다고 판단된다.
CERVUS ver.3.0.7을 사용한 maternity analysis 결과(Table 5), critical delta 값은 95% 신뢰수준과 80% 신뢰수준 모두에서 0.00이었다. 95% 신뢰수준과 80% 신뢰수준에서 모수가 충북2호 또는 강원40호인 개체는 총 81개(77.1%)였다. 가장 가능성이 높은 후보 모수와 유전자형의 불일치가 있는 3개(2.9%)의 차대목들은 모두 모수가 충북2호도, 강원40호도 아닌 것으로 나타났다. 강원40호가 모수일 가능성이 가장 높은 개체 중 21개(20.2%)의 차대목들도 강원40호가 모수가 아닌 것으로 식별되었다. 모수가 충북2호 또는 강원40호로 밝혀진 차대목들은 모수와 유전자형 불일치가 없었다.
강원40호와 유전자형의 불일치가 없지만 통계분석에서는 강원40호가 모수가 아닌 것으로 나타난 21개체의 경우 위음성(false-negative)일 가능성이 있다. 예를 들어, 1차 분석에서 충북2호와 강원40호가 모수가 아닌 것으로 확인된 8개 개체(3-45-10a, 3-45-11a, 3-50-2, 3-51-7, 3-52-5, 3-53-8, 3-53-9, 3-54-10)는 2차 분석에서 모수가 강원40호로 나타났다. 분석 간 식별 결과의 차이는 1차 분석에서 모수가 지정된 경우에도 나타난다. 1차 분석에서 충북2호가 모수인 것으로 나타난 개체 중 세 개체(3-44-11a, 3-47-7a, 3-53-7)는 2차 분석에서 강원40호가 모수인 것으로 나타났다.
2차 분석에서 모수가 충북2호나 강원40호로 식별된 차대묘들만 이용하여 2차 parent pair 분석을 실시했다(Table 6). Critical delta 값은 95% 신뢰수준에서 2.20 그리고 80% 신뢰수준에서 0.00이었다. 81개 개체 중 47개(58.0%)가 충북2호와 강원40호의 친자 혈통으로 식별되었다. 또한 3개체(2.9%)가 충북 2호의 자배로 만들어진 개체로 추정되었으며, 2개체(1.9%)가 강원40호의 자배로 만들어진 개체로 추정되었다. 친자 혈통, 충북2호의 자배 및 강원40호의 자배로 만들어진 것으로 식별된 차대목들 모두 부모와의 유전자형 불일치가 없었다.
The values in bracket are about all samples, the values out of bracket don’t contain samples which mother tree is neither CB2 nor KW40.
두 번째 parent pair analysis 결과(Figure 2) LOD score가 0을 넘는 차대묘는 47개였으며 LOD score가 0이하인 차대묘는 34개였다. 이 때 LOD score가 0을 넘는 차대묘들 모두 delta 값이 0을 넘었다.
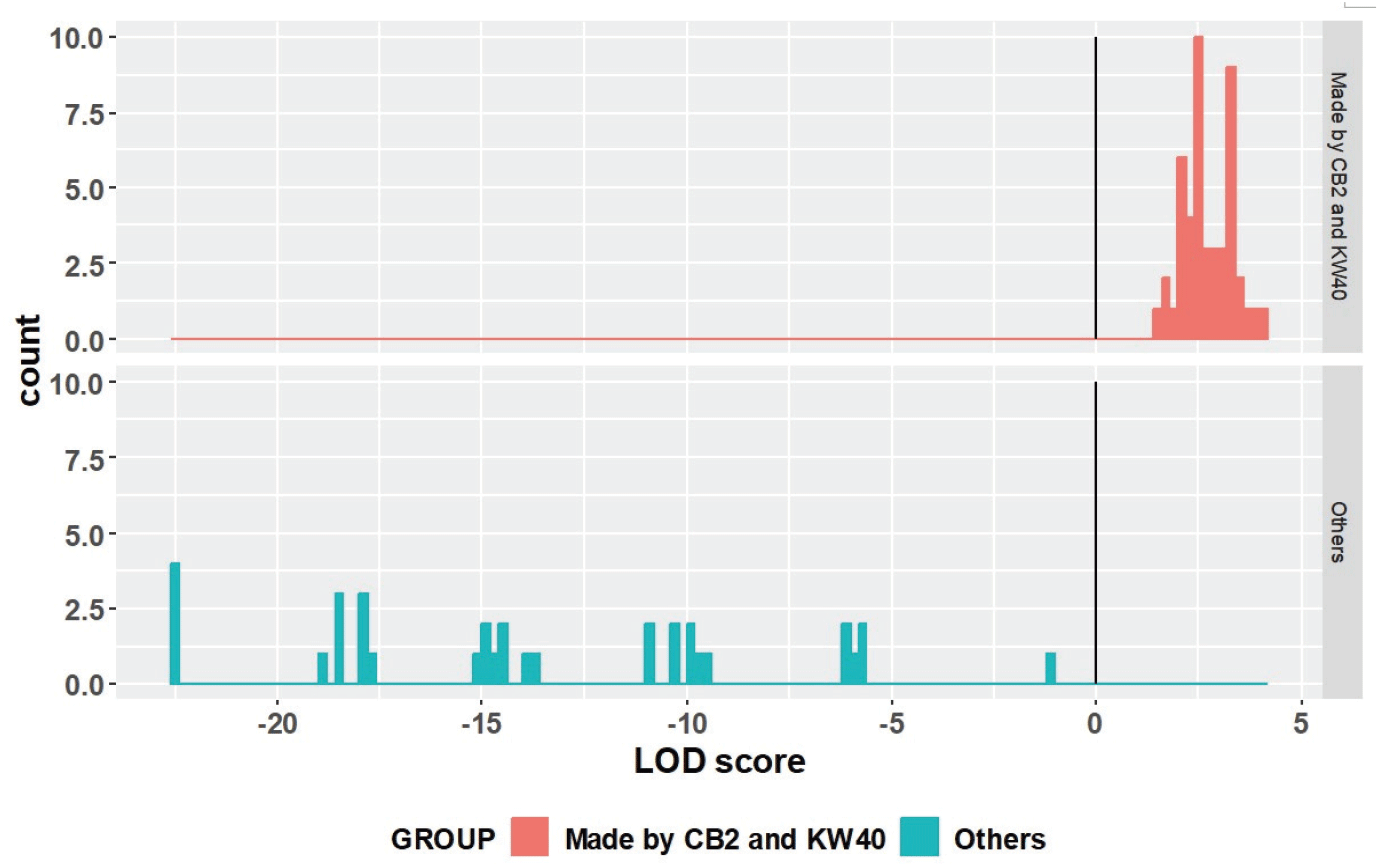
2차 분석에서도 1차 분석과 마찬가지로, 소집단 1의 차대목 모수가 강원40호로 식별되는 문제가 존재했다. 소집단 2에서 중복된 인식표를 가지는 개체가 같은 모수를 가지는 문제 역시 존재했다. 인식표가 3-47-8이었던 세 개체는 2차 분석에 사용되지 않았으므로 세 개체가 같은 인식표를 가지는 문제점은 없었다. 이와 같은 개체들을 제외하면, 전체 159개 차대묘 중 68개체(42.8%)가 관리상의 문제가 의심되지 않으면서, 충북2호 또는 강원 40호가 모수로 식별되었다. 다만, 모수 후보목과 유전자형의 불일치가 존재하는 차대목은 전체 159본 중 3본(1.9%)에 불과했다. 또한, 친자 혈통이라고 판정된 차대묘 중 관리상의 문제가 의심되지 않는 개체는 전체 159개 차대묘 중 44개체(27.7%)였다. 본 연구의 인공교배 효율은 27.7%로 판단된다.
본 연구의 인공교배 효율을 선행연구와 비교하기 위해서, 앞선 연구결과들을 제시하고자 한다. 선행연구에서는 인공교배 기록과 microsatellite 분석을 이용한 모수, 화분수 확인 결과를 비교하여 인공교배 효율을 결정했다. 잎갈나무 채종원의 경우 모수를 기준으로 할 때의 효율은 47.9% (123/257), 화분수를 기준으로 할 때의 효율은 43.6% (112/257)였다(Sun et al., 2017). 테다소나무 채종원을 대상으로 한 연구에서는 2005년의 인공교배와 2006년의 인공교배의 효율을 평가했다. 2005년의 인공교배 효율은 모수를 기준으로 할 때 56.9%(91/160), 모수와 화분수를 모두 고려했을 때 21.3%(34/160)이며 2006년의 인공교배 효율은 모수를 기준으로 할 때 80.0%(112/140), 모수와 화분수를 모두 고려했을 때 67.1%(94/140)였다(Grattapaglia et al., 2014). Kumar et al.(2007)의 라디아타소나무 연구에서는 모수를 기준으로 했을 때의 인공교배 효율이 72.1%(165/229)였다.
본 연구에서 충북2또는 강원40호가 모수이며, 관리상의 문제가 의심되지 않는 차대목의 비율(42.8%)은 Sun et al.(2017)의 연구와 비슷한 값이라고 할 수 있지만, 다른 연구에 비해서는 낮은 값을 나타낸다. 본 연구에서의 인공교배 효율(27.7%)은 모수와 화분수를 모두 고려한 2005년 테다소나무 채종원 인공교배 효율(21.3%)과(Grattapaglia et al., 2014) 비슷하지만 다른 수치들에 비해서는 낮은 값을 보인다.
이 때, Sun et al.(2017)의 연구에서 모든 교배조합이 인공교배 효율이 높은 것은 아니다. 잎갈나무 교배조합 중 59×120이 가장 극단적인 예를 보여주는데, 59×120의 차대목 7개체 중 모수가 59인 개체는 없었으며(0.0%), 한 차대목의 화분수만 120으로 식별되었다(14.3%). 77×98의 경우 총 10개의 차대목 중 모수가 77인 개체는 5개였으며(50.0%) 화분수가 98인 개체는 2개체 존재했다(20.0%). 이를 고려한다면, 인공교배를 실시할 때 낮은 인공교배 효율이 나타날 가능성이 존재한다고 생각한다. 본 연구와 유사한 결과값의 발생 가능성도 충분히 존재한다.
본 연구의 결과를 네 가지로 진단해볼 수 있다. 먼저, 채종원 조성 당시 채종목의 인식표가 잘못되었을 가능성이 존재한다. 모수로 사용된 채종목의 클론명이 잘못 기재된 경우 통계분석에 의해 식별된 인공교배 차대묘들의 모수가 인공교배 기록상의 모수와 달라질 수 있다. Grattapaglia et al.(2014)은 채종목 인식표 오류를 지적했다. 테다소나무 연구대상 교배집단 중 F05_1(2005년 인공교배) 집단은 모수가 101로 식별되어야 하지만 F05_1인공교배 집단에서 차대목 중 90% 이상의 모수가 103으로 식별되었다. 모수로 사용된 채종목의 인식표 오류에 의해 이와 같은 문제가 발생할 수 있다. 본 연구에서도 차대묘 인식표를 바탕으로 한 예상과 통계분석 결과가 다른 차대목이 총 64개체(40.3%, 관리 문제 가능성이 존재하는 67개체 중 관리 문제가 분명한 세 개체(3-47-8a, 3-47-8b, 3-47-8c) 제외) 존재했다. 본 연구에서는 충북2호, 강원40호의 유전자형만을 이용하여 분석을 했지만, 모수 식별에서의 문제가 있는 64개체가 모수로 사용된 채종목의 인식표 오류에 의해 만들어졌을 가능성이 있다.
화분오염에 의해서 화분수 식별 결과와 인공교배 기록상의 화분수가 다른 인공교배 차대목이 만들어질 수 있다. Sun et al.(2017)은 잎갈나무 인공교배 시 화분오염이 있었음을 지적했는데, 채종원 내 임목의 화분과 채종원 외부 임목의 화분 모두가 화분오염을 일으켰다. Adams et al. (1988)은 화분오염이 일어나는 원인들을 제시한다. 미숙한 화분 채취 기술, 의도치 않은 화분 혼합이 화분오염을 일으킬 수 있다. 인공교배를 하는 동안 교배조합의 화분수가 아닌 다른 임목에서 온 화분을 주입하는 경우에도 화분오염이 일어날 수 있다. 또한, 암구과의 격리도 화분오염을 막는데 중요한 요소이다. Grattapaglia et al.(2014)은 2005년에는 수분을 위해 교배봉투를 잠시 제거해야 했지만, 2006년에는 주사기로 화분을 주입할 수 있는 교배봉투를 사용했다고 밝혔다. 결과적으로 이러한 차이가 인공교배 성공률을 높였다. Kumar et al.(2007)은 화분을 채취하는 시기에 연구자가 사용하는 화분수 외에 다른 임목에서도 화분이 비산되는 것도 문제라고 언급했다.
본 연구에서는 화분오염 가능성이 존재한다. 앞서 밝힌 대로, 교배를 할 때 고온으로 인해 교배봉투 적용 시기를 예측하기가 어려웠으며, 강풍으로 인해 손상된 교배봉투도 존재했다. 이러한 문제 때문에 화분오염이 발생했을 수 있다. 또한 화분 비산 시기의 동일성 때문에, 인공교배를 위해 채취된 화분에 충북2호와 강원40호 외의 채종목에서 비산된 화분이 섞여 있을 가능성을 배제할 수는 없다.
화분오염의 원인에는 인공교배 기법과 무관한 요인도 존재한다. Adams et al.(1988)은 의도치 않은 화분 오염의 주요 원인 중 하나가 채종원 내 채종목의 인식표 오류라고 지적한다. Grattapaglia et al.(2014)역시 화분수로 사용된 채종목의 인식표 오류가 화분수 식별 오류를 만들어 냈을 가능성도 제기하는데, 이는 인식표에 문제가 있는 채종목에서 화분을 채취했기 때문이다. 2005년의 테다소나무 인공교배에서 103, 107클론 등이 모수와 화분수로 모두 사용되었는데, 본 연구의 충북2호와 강원40호도 모수와 화분수로 모두 사용되었다. 따라서 본 연구에서 모수의 채종목 인식표 오류가 존재했다면, 인식표 오류가 있는 채종목에서 화분이 채취되었을 가능성이 존재한다. 이에 따라 화분수 인식표 오류에 의한 화분 오염이 나타날 수 있다.
차대묘 인식표를 바탕으로 한 예상과, 통계분석 결과가 다른 문제는 교배조합 차대묘 관리 문제 때문에 발생했을 수도 있다. 본 실험의 실험 대상은 두 개의 교배조합이 혼합된 집단이었다. 즉, 차대묘를 관리하는 과정에서 충북2호×강원40호 집단과 강원40호×충북2호 집단이 제대로 분리되어 있지 않았다. 3-47-8의 경우 동일한 인식표를 가지는 개체가 세 개체가 있었다. 따라서 두 집단이 섞이는 문제 외에 다른 관리상의 문제 가능성도 존재한다. 집단을 조성할 때 충북2호×강원40호, 강원40호×충북2호 외에 충북2호 풍매 집단, 강원40호 풍매 집단도 같이 조성했는데, 풍매 개체가 인공교배 차대묘 집단에 섞여 있는 경우 문제를 야기할 수 있다.
마지막으로 구과 채취와 종자 처리에서의 문제가 존재할 수 있다. Kumar et al.(2007)은 인공교배 후 구과 채취 과정에서 풍매 구과를 채취하는 경우 인공교배 결과에 문제가 생길 수 있다고 지적한다. Grattapaglia et al.(2014)은 교배조합 간 종자가 섞이는 문제를 배제하지는 않으며 Sun et al.(2017)은 종자를 수집할 때 주의가 필요하다고 주장한다. 본 연구에서도 구과 채취부터 파종 단계까지 종자가 섞였을 가능성을 배제할 수는 없다.
본 연구의 인공교배 효율이 높다고 판단할 수는 없지만, 인공교배 차대묘의 활용 가능성에 대해서 따로 고찰할 필요가 있다. 2005년에 실시된 테다소나무 인공교배에서 88.1%(141/160)의 차대묘의 모수가 인공교배에 사용된 채종목으로 식별되었다. 또한, 모수와 화분수가 모두 인공교배에 사용된 채종목인 차대묘의 비율은 64.4%(103/160)였다. 물론, 2005년 당시의 인공교배 기록을 기준으로 계산된 인공교배 효율은 모수를 기준으로 했을 때 56.9%, 모수와 화분수를 모두 고려했을 때 21.3%였다. 하지만 해당 연구를 수행한 학자들은 통계분석에 따른 모수를 기준으로 인공교배 차대묘 집단의 활용 가능성을 고찰했다. 해당 연구진은 채종목을 모수와 화분수로 둔 차대묘는 유전적으로 우수하다고 판단하였고, 이를 근거로 해당 연구자들은 2005년에 만들어진 인공교배 차대묘가 조림에 사용될 수 있다고 주장했다(Grattapaglia et al., 2017).
차대묘 인식표와 무관하게, 본 연구에서는 134본의 차대묘(84.3%, 1차 분석에서 모수가 식별된 126본, 1차 분석에서는 모수가 지정되지 않았지만 2차 분석에서 모수가 식별된 8본)가 충북2호 또는 강원40호를 모수로 두고 있다는 결과가 나타났다. 또한, 총 156본(98.1%)의 차대묘가 해당 차대묘의 가장 가능성이 높은 모수와 유전자형 불일치가 없었다. 통계 분석만을 기준으로 판단했을 때, 60본의 차대묘(37.7%)가 충북2호와 강원40호의 교배로 만들어졌다. 37.7%라는 수치가 Grattapaglia et al.(2017)이 제시한 64.4%보다는 낮지만, 모수를 기준으로 했을 때의 비율이 비슷하므로 본 연구의 인공교배 차대묘 집단도 조림용으로는 사용이 가능할 수 있다. 물론 더 많은 유전획득량을 얻기 위해서는 더 높은 인공교배 효율이 필요하다. 또한, 충북2호와 강원40호의 친자 혈통만 사용할 수 있는 유전학 연구도 존재하므로, 이러한 연구를 위해서도 더 높은 인공교배 효율이 필요하다.
Microsatellite를 이용하여 충북2호×강원40호와 강원40호×충북2호가 섞인 집단에 대한 혈통분석을 진행하고, 인공교배 기록과 상충하는 결과들을 배제함으로써 42.8%의 모수 일치율과 27.7%의 인공교배 효율을 확인할 수 있었다. 이는 선행연구의 인공교배 효율보다 낮은 수치였다. 이러한 문제는 모수로 사용된 채종목의 인식표 문제, 화분오염 문제, 구과 채취 및 종자 관리의 문제와 교배 차대묘 관리 문제에 의해 발생할 수 있다. 이를 해결하기 위해서 채종원 내 채종목의 인식표 정확성 분석, 화분오염을 막을 수 있는 인공교배 기법 사용 및 구과와 종자 처리 시 실수를 줄이는 방법이 요구된다. 선행논문과 달리 이번 연구에서 차대묘 집단 간 분리를 포함하는 차대묘 관리 문제를 제기할 수 있었다. 따라서 파종 이후의 올바른 차대묘 관리도 인공교배의 정확도를 높이기 위해 중요하다. 인공교배를 통해 만들어진 차대묘들은 수목의 유전학 연구, 교잡육종 등에 사용된다. 또한 인공교배 차대묘는 진전세대 채종원을 조성하는데 사용되며, 조성에 사용된 인공교배 차대묘들이 생산한 종자에 의해 조림이 실시된다. 이러한 인공교배의 활용을 고려한다면 높은 인공교배 효율은 여러 사업과 연구가 효율적으로 진행되기 위해 요구되는 조건이라고 할 수 있다. 따라서 인공교배가 성공적으로 될 수 있도록 앞에서 지적된 네 가지 사항에 주의하여 인공교배를 진행해야 한다.